In the constantly changing technology of artificial intelligence Gender-specific trained Transformers (GPT) model are in the top of the line. They are used in a variety of ways that range from writing articles and composing documents, to answering questions, creating concepts, and even having conversations that feel like human. But, even though GPT models have impressive capabilities however, they aren’t without limits. To better understand their limitations and make the most of them Let’s look at the top 5 limitations for GPT models.
1. Lack of True Understanding and Contextual Awareness
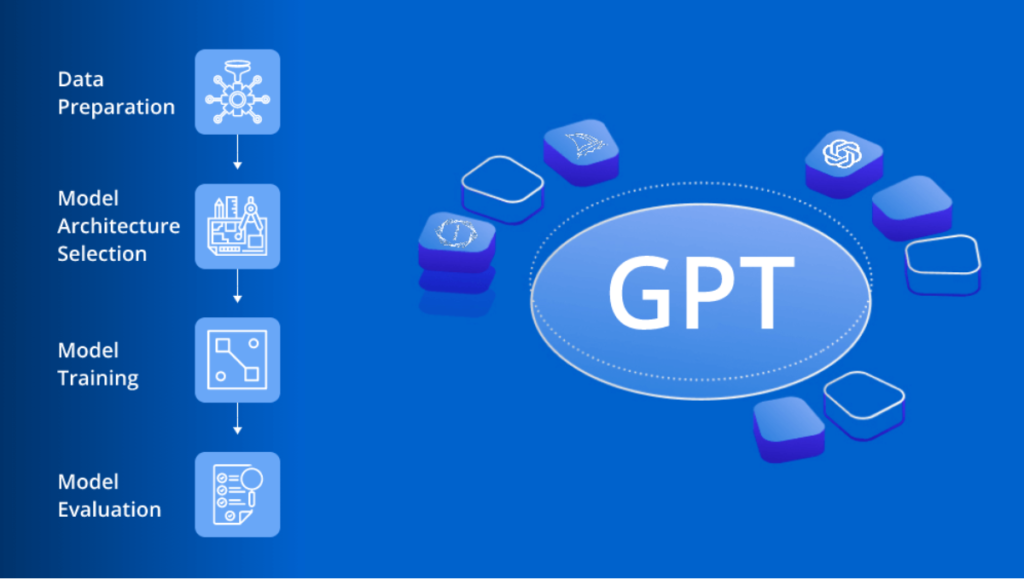
GPT models regardless of their size or sophistication, function by analyzing patterns derived from huge amounts of text. They do not “understand” text in the same way that humans do. They can predict the meaning of words and phrases on their knowledge of statistical patterns, but without a fundamental knowledge of the ideas that are involved.
- Consequences of Limited Understanding
This issue often payoff in outputs that sound plausible but aren’t relevant or deep for specific situations. For instance, when asked a difficult question that requires more nuanced understanding and nuanced understanding, GPT models GPT model could produce words that sound coherent, however it is in reality superficial or fails to capture the core of. - Examples in Practice
Imagine a scenario in which models provide suggestions on financial or medical issues. The model’s response may not be able to provide the knowledge or understanding that an experienced competent can bring to the discussion. The absence in “true understanding” can be crucial in areas that require contextual and factual accuracy. is vital.
2. Inability to Access Real-Time Information
GPT models are restricted to the data they were trained on. they were trained, and up to a particular cutoff date. They cannot retrieve or access live updates, current events or information that is dynamically changing without particular integrations or plugins.
- Impact of Outdated Information
For topics such as recent events and stock market news or advances in technology and medical technology, GPT models can help in providing only historical views. This is particularly problematic when people are looking for the latest developments in rapidly-changing fields. - Potential Workarounds
To compensate, users could require more devices or input manually information manually. For instance, some applications are integrating GPT models that use internet access or APIs that get real-time data, however this solution is in the beginning stages and will require additional the complexity.
3. Prone to Generate Biased or Inappropriate Content
GPT models are influenced by the data they’re taught from that is primarily sourced via the internet. Because human-authored texts are subject to biases or stereotypes as well as particular cultural views, GPT models can unintentionally duplicate and even amplify the biases they encounter in responding.
- Risks of Bias in Various Applications
In skillful applications, such as recruitment or material production, this may produce biased outputs that discriminate against specific groups or spread negative stereotypes. Furthermore, if not used with adequate control, these biases may influence decisions or interactions with customers in non-intentional ways. - Challenges in Bias Mitigation
The developers have efforts to solve this problem through fine-tuning models as well as filtering out harmful material. But, completely eliminating biases is a difficult task because of the variety and quantity of the information on that GPT models are based.
4. Vulnerable to Misinformation and Fabrication
Top 5 limitations of GPT models aren’t perfect judges of truth. They create text based on patterns, rather than facts that have been verified, so they could produce incorrect or false data. This tendency for “hallucinate” facts can be difficult in situations in which accuracy is essential.
- Example of Hallucination in Material Generation
Imagine a client wants to ask the GPT model for specific information about an obscure topic. The model may give plausible but fake information, if it’s not backed by pertinent information, since it puts coherence ahead of accuracy. The resulting “hallucinations” can be hard to discern for those who aren’t experts on the area. - Implications for Users and Developers
This restriction requires fact-checking especially in fields like healthcare or law where misinformation could have devastating negative consequences. For GPT model applications that require precision, the developers might need to integrate the model with other sources or establish rigorous verification procedures.
5. Limited Problem-Solving and Reasoning Abilities
Although GPT models excel at producing text and processing however, they are often challenged by tasks which require logical reasoning complex problem-solving and multi-step thinking. These types of tasks are beyond the responses based on patterns GPT is designed to generate.
- Examples in Logical and Analytical Scenarios
When solving math-related questions such as riddles, multi-step questions, GPT models may produce inaccurate solutions or fail keep the same consistency over various levels. The model could give an answer that is like it is coherent, but does not stand when analyzed for the consistency of logic or accuracy. - Limitations in resolution-Making Applications
When it comes to tasks which require critical thinking — such as decision-making assistance, in-depth analysis of data, or strategic planning, models from GPT tend to be inadequate. They do not have the capacity to weigh the pros and cons, evaluate the risk, or make a decision from experience, all factors which are essential for precise decision-making.
The Road Ahead: Balancing Limitations and Opportunities
Despite their limitations GPT models have certainly changed the field of natural processing of language as well as AI applications. These models excel at tasks that require the generation of language or pattern identification. Especially when they are used for creativity in writing automated customer service, material generation, and conversational AI. When accuracy, relevance in real time and impartial responses are not a given the user must be wary. And think about combining GPT by together other methods of verification, or with expert input.
Current research seeks to overcome these shortcomings by creating models. They are more intelligent and enhanced bias control and the ability to connect with information in real-time. As AI technology develops, the goal is to build AI systems that don’t just replicate human language patterns. But additionally interact with data in ways that allow them to be closer to human comprehension, reasoning and aptitude.
In summary, GPT models are powerful but flawed tools. They perform desirable when people are aware of their limitations and augment their capabilities with oversight by humans as well as validation. And where necessary, extra resources. In this way we can draw the advantages of these models. While being alert to their weaknesses. And pave the way for the future in which AI assists, not directs our interactions with data.