Artificial Intelligence (AI) is transforming the world at a rapid pace. As AI systems become more sophisticated, ensuring fairness becomes essential. We will discuss the principle of fairness in Gen AI Entail
In simple terms, the principle of fairness in Gen AI is about creating systems that make unbiased decisions and treat everyone equally, regardless of factors like race, gender, or background. These systems should base their decisions on relevant data, not on discriminatory or irrelevant characteristics.
In this blog, we’ll explore the importance of fairness in AI, the challenges it poses, and how we can build AI systems that are more inclusive and equitable. Let’s dive into this crucial topic!
Table of Contents
- What is Fairness in Gen AI?
- Principles of Fairness in Gen AI
- Non-discrimination
- Inclusivity
- Transparency
- Accountability
- Common Challenges to Fairness in Gen AI
- Implementing Fairness in Gen AI
- Use Diverse Data
- Test for Bias
- Build Diverse Teams
- Make AI Explainable
- Set Clear Fairness Goals
- Keep Learning and Improving
- Real-World Examples of Fairness Issues in Gen AI
- Conclusion
What is Fairness in Gen AI?
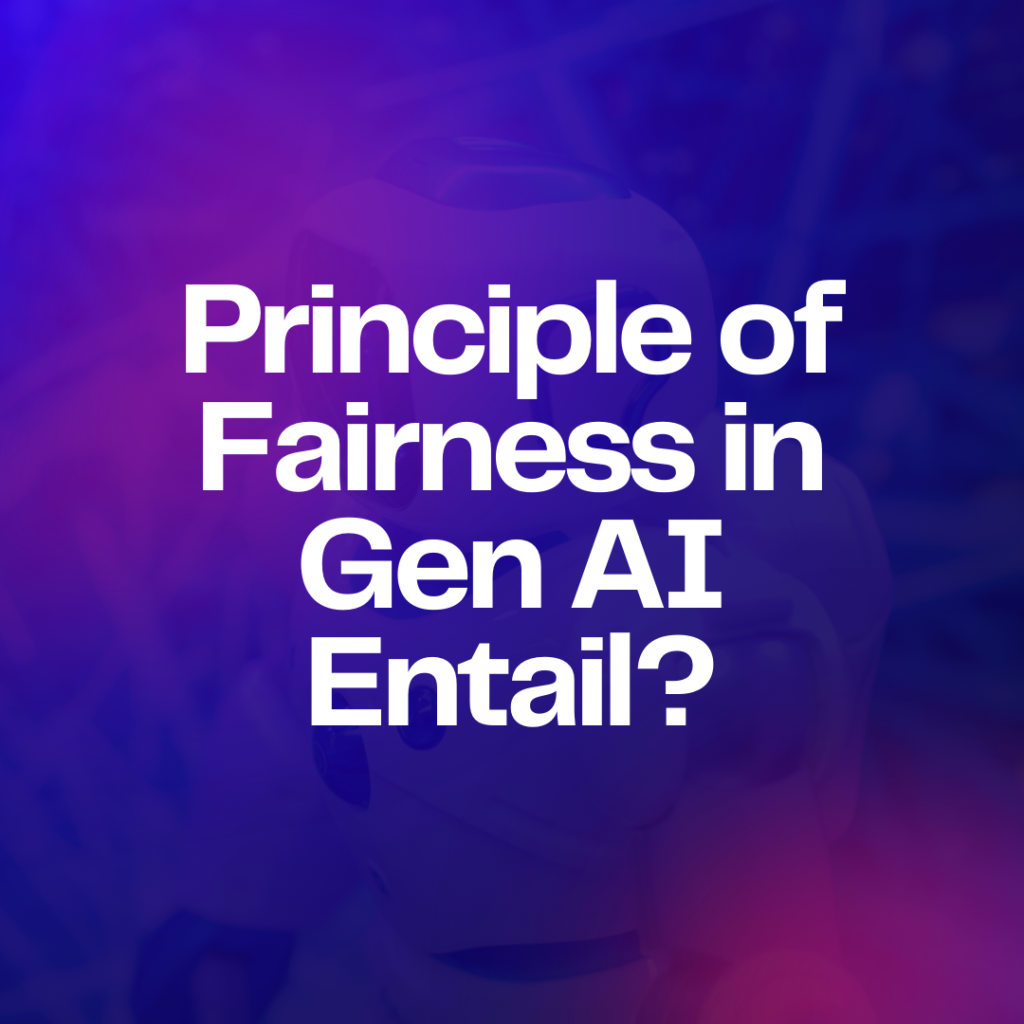
Fairness in General AI means designing intelligent systems that treat all individuals equally and justly. Like a fair referee in a sports game, AI should make decisions based solely on relevant data and facts, not on any biases related to a person’s race, gender, or background.
In many fields—such as job recruitment, loan approvals, and law enforcement—AI plays a growing role in decision-making. Therefore, ensuring fairness in these systems is vital for creating a just and equitable society where everyone has the same opportunities.
Principle of Fairness in Gen AI Entail
Several core principles guide the implementation of fairness in AI systems:
1. Non-discrimination
AI systems should not discriminate against individuals based on protected characteristics such as race, gender, age, or socioeconomic status. Decisions must be made based on relevant and justifiable factors only, without any hidden or implicit biases.
2. Inclusivity
Inclusivity ensures that AI systems are designed to serve all groups of people. This requires considering diverse perspectives and needs throughout the AI development process to avoid creating systems that only work well for specific demographics.
3. Transparency
The decision-making process of AI systems should be clear and understandable. Users need to know how and why the AI made a particular choice, especially in critical areas like hiring, healthcare, or legal decisions.
4. Accountability
There should be mechanisms to monitor and evaluate AI systems for fairness. If any problems arise, there should be clear processes in place to address and rectify them.
Common Challenges to Fairness in Gen AI
Achieving fairness in AI systems is not without its challenges. Some of the key obstacles include:
- Biased Data: AI systems often learn from historical data that may be biased. If the data used to train an AI model contains patterns of discrimination, the AI may perpetuate or even amplify these biases.
- Unconscious Bias: The people developing AI systems may unknowingly introduce their own biases into the design, leading to AI that favors certain groups over others.
- Complex Decision-Making: AI models can be complex and opaque, making it difficult to understand or explain why they made a particular decision, thus making it harder to detect unfair outcomes.
- Balancing Competing Goals: Ensuring fairness can sometimes conflict with other objectives, such as optimizing for accuracy or efficiency, making it a challenging balancing act.
Implementing Fairness in Gen AI
To address these challenges, several strategies can help make AI systems more fair:
1. Use Diverse Data
AI systems should be trained on diverse and representative datasets. This ensures that the system doesn’t favor one group over another and that it performs equally well for all people.
2. Test for Bias
Regularly testing AI models for bias is crucial. Tools and techniques can be used to detect hidden biases and correct them before the system is deployed.
3. Build Diverse Teams
Diverse teams, including individuals from different backgrounds, bring varied perspectives to AI development. This diversity helps identify potential issues and build systems that are more inclusive.
4. Make AI Explainable
AI systems should be designed to explain their decisions in a way that users can understand. This transparency builds trust and allows people to hold AI systems accountable for their decisions.
5. Set Clear Fairness Goals
Clearly defining what fairness means for each AI system is essential. Setting measurable fairness goals allows developers to track how well the system is meeting these objectives and identify areas for improvement.
6. Keep Learning and Improving
Fairness in AI is an evolving field. Staying updated on the latest research and techniques, and continuously improving AI systems, ensures they remain fair as new challenges arise.
Real-World Examples of Fairness Issues in Gen AI
Here are a few real-world cases where fairness in AI came into question:
1. Facial Recognition Problems
Some facial recognition systems have been found to have trouble identifying people with darker skin tones. These biases have led to false matches and unfair outcomes, prompting companies to refine their models to improve accuracy for all skin tones.
2. Job Application Screening
An AI system used by a major company to screen job applications was found to favor male applicants over female ones. This bias was a result of the AI learning from historical data where more men were hired. The company had to revise the system to ensure equal consideration for all candidates.
3. Loan Approval Bias
In some cases, AI systems used for loan approvals have been found to grant lower credit limits to women, even when their financial qualifications were similar to men’s. This highlighted the need for fairness checks in financial decision-making systems.
Conclusion
Principle of Fairness in Gen AI Entail is critical for creating a future where technology works for everyone equally. By ensuring that AI systems are non-discriminatory, inclusive, transparent, and accountable, we can avoid perpetuating existing biases and injustices.
While challenges like biased data and unconscious prejudice make achieving fairness difficult, there are clear strategies we can employ to build fairer AI systems. Collecting diverse data, testing for bias, and fostering inclusive development teams are all crucial steps in this direction.
Ultimately, fairness in AI is not just a technical concern—it directly impacts people’s lives. As AI becomes more integrated into our daily routines, it’s up to all of us to push for systems that uphold the principle of fairness, ensuring a just and equitable future for all.